While nearly all the companies know
that their data is a strategic asset, most of them are not taking full benefit
of big
data analytics to get ahead. This post explains the key elements of a successful
data strategy which will assist you make decisions on the basis of right data
analysis instead of hunches.
Companies know that their product
data is a strategic asset and plan to use it to make better decisions, but the issue
is that it is complicated. Usually, the data gets distributed in silos, caught
in departmental systems which don’t work well with each other, the data quality
is poor and costs related to it are really high. Besides handling the market
pressures, most companies will prioritize the critical, strategical and routine
requirements over the long-term strategic initiatives.
Heading towards a totally
data-driven culture is definitely possible and it begins with a powerful and
effective big data strategy, which usually seen as a technical practice, but a
modern and broad data strategy addresses more than just data and is a roadmap which
defines the people, process and technology for proper big
data analytics.
An effective data strategy must outline
a detailed plan to evolve in analytic abilities and evolution from making
decisions on the basis of observation to make decisions with prudence.
Let’s know now how you can get
started with your own effective data strategy.
Take a Look at a Few
Important Elements of a Successful Big Data Analytics Strategy:
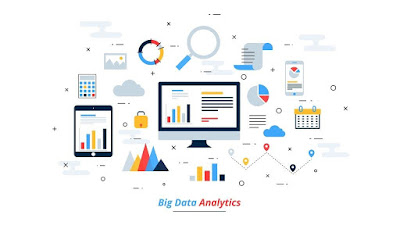
1. Business Needs:
Data has to address particular business needs for meeting the company’s
strategic objectives and generate actual value out of it. The first step to set
the business needs is to recognize a winner, all stakeholders and SMEs in the company.
The winner of the data strategy is the executive leader that will gather the support
for the investment. Stakeholders and SMEs will represent certain departments or
functions in the company. After that companies must set the strategic objectives
and integrate department activities to company objectives. It is obvious to
have company objectives and department level objectives, but the stated objectives
for both levels must be synchronized. These goals are most efficiently collected
through an interview process which begins at the executive level and then continues
down towards the department leaders. With this process, you can find out what the
leaders are trying to asses and improve, questions they need answers for and
then the KPIs to answer those questions. Through beginning with collecting and
documenting the business needs, companies overcome the first obstruction to
many IT or technical projects- knowledge of what the business is trying to
accomplish.
2. Sourcing
and Collecting the Data: With proper understanding of what
questions exactly the business is asking, companies can shift to the next
element- analyzing data sources, in what ways that data is collected and where
exactly the data really exists. It is doubtful that all company data is
available in the company and that it already available in a place which is accessible.
So, companies should work backwards to find out the source. For data which can
be found in-house, your
big data
analytics team must note that the source system and any hurdles to getting access
to this data. Make sure that you find out whether the data has the correct
level of detail and is updated with the correct frequency to reply back to the
question successfully.
3. Technology
Infrastructure Needs: Never get over-involved in the buildup and
the latest technologies, rather concentrate on the commercial reasons for your
initiatives. Establishing a flexible data architecture is a complex subject for
which there are several options and strategies, so below mentioned are a few vital
things to consider:-
- To what degree can an existing operational
system support big data analyticsrequirements?
Well, very little. It is usually not the most excellent approach to depend on
an operational system to fulfill analytical requirements that means a central
data repository can be very useful.
- Whether your company has the ability and
technical infrastructure to support a data warehouse on-premise or would it
leverage a big
data in cloud solution, think what makes more sense.
- Find out whether there is a standard integration
tool to access the data through the source systems in the central repository or
not. Will this architecture layer get leveraged for commercial logic, so the
data is all set to be used?
All of the above
mentioned considerations will get within an overall architecture and as with nearly
all designs, the more your needs and future requirements are considered, the
more the big data analytics solution will really support the business.
4. Transform
the Data into Insights: A data strategy must offer suggestions
for how to apply
big
data analytics to dig out business-critical insights, and big data
visualization is the key! A lot of new-age companies still depend on Excel, e-mail
or an inheritant business intelligence tool that do not allow to communicate
with the data. Often a boring and manual process is needed and depending on IT
to build reports makes a blockage. Data visualization tools must make the data
look excellent and make the data simpler to understand.
5. Processes
And People Involved: In this step companies monitor the people within
their company and the processes connected with building, sharing and ruling
data. A data strategy will possibly introduce more data and big data analysis
and even new tools. On the basis of this, it looks sensible enough to look at
the talents of the users to comprehend their strengths and where exactly they
might need support. Employee feedbacks and incentive strategies must be assessed.
This can further be used as levers to motivate employees to make use of data in
the way company aims. While employees are provided new tools, but not shown the
right approach to think in a different way about their jobs, the outcome won’t
really change. Speaking about the process, several companies have unplanned barriers
to make use of their data for decision making. Business processes might require
being re-engineered to integrate
big
data analytics. This can be accomplished through documenting the steps in a
process and where certain reports get leveraged for one decision. Recognition would
go a really long way as you win on the basis of new use of data, it must be rejoiced
and endorsed to create internal drive and motivate positive behaviors with
data.
6. Data
Governance: Data governance eventually allows the company level
sharing of data and the fuel that lubricates the functioning of a big data
analytics practice. A data governance plan will make sure that computations
used across the company are decided on the basis of input from across the company,
the correct people have access to the right data and data lineage is set. Companies
do not really look to a tool for solving data governance, as it is people work
and it must happen. Data governance guides the way and often navigates through complex
interactions. Building a data dictionary is a great place to begin with. A data
dictionary is an important document wherein all available end-user evaluations
and dimensions are officially described. In these interactions and misinterpretations
regarding terms are recognized and corrected.
7. Final
Strategy: The final strategy is the combination of all the work an
organization have done to this point and what makes all their previous work doable.
Companies have identified all that requires to happen to make you reach your
destination, but prior to beginning with any design, develop, training or re-creation
of a business process, it is very important to prioritize the activities. For every
suggestion that will assist bridge the gap from existing position to the future
position, describe the feasibility and projected business value it will offer.
The strategy must prioritize activities which are simplest to execute, but also
offer fast success to the business. Other factors must include in the final
strategy are staff availability or exterior assistance is needed, company’s
budgeting process and a timeline which enables for celebration of incremental successes
earned along the way.
So, encompass these elements of a
successful big
data analytics strategy to manage your company data effectively.
No comments:
Post a Comment